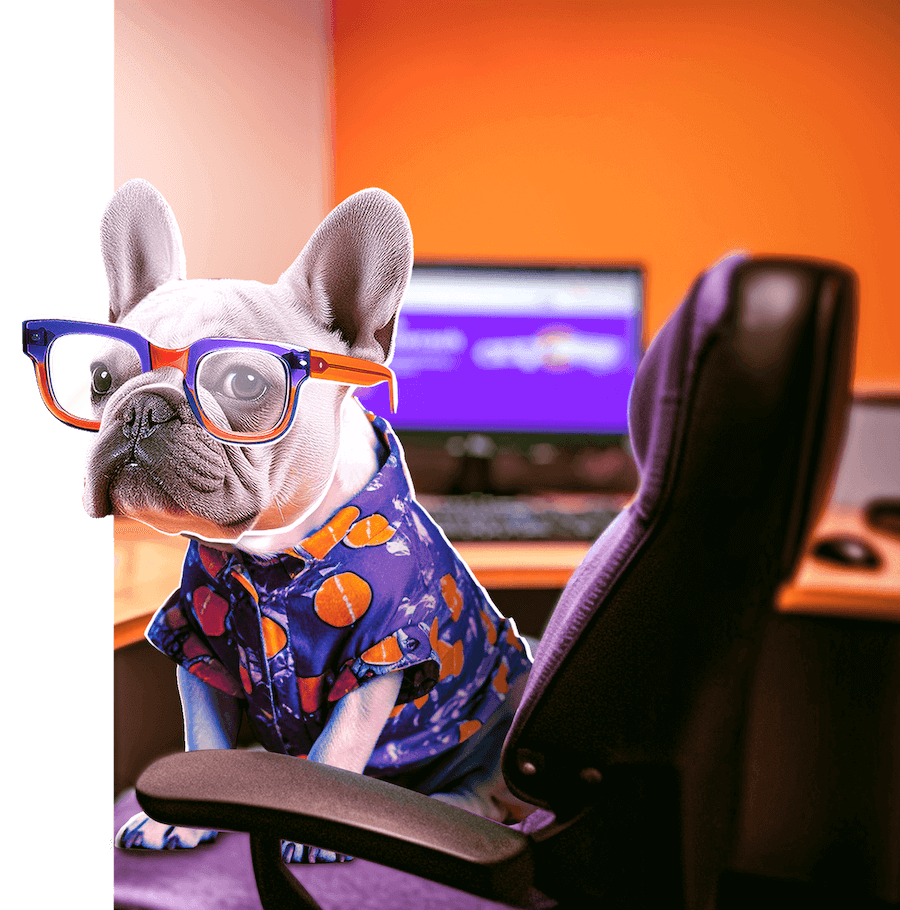
Demographic Profile
Comment
Technology
Comment
Comparing the latest report with that of this year, it's evident that Machine Learning (ML) has become more than just technology - it is now the basis of innovation in companies, firmly confirming its place in the market with an impressive 83.7% adoption rate. It's as if ML has entered the mainstream of business, becoming a fixed point in development plans. Last year's data showed a stronger attachment to traditional techniques like Statistical Analysis and Supervised Learning. Meanwhile, Deep Learning has gained momentum and is now used in increasingly difficult data tasks - it's somewhat like an upgrade in the world of AI. Interestingly, the great expectations for NLP, which were a hit last year, have not been fully met, but instead, new, more specialized methods like Fine-tuning LLM and Transfer Learning are emerging. Particularly the latter is now coming into the limelight as a method for transferring experience between different types of tasks. In short, data science is moving towards more bespoke techniques. This shows how the industry can adapt and evolve to meet new technological and business challenges, giving us a taste of how it may change in the coming years.
Comment
Working with data according to the Pareto principle means spending 80% of the time on reviewing, cleaning, and analyzing data. Python is most commonly used for data analysis, so it's no surprise that Pandas, Jupyter Notebook, and the SciKit Learn package are at the forefront. These are the three fundamental (and often sufficient) elements for preparing even complex analyses or ML models.
Excel is used by almost half of the respondents, as it is known that Excel is the king of corporations and spreadsheets. Its advantage is that many things can be done quickly in it, without writing even a line of code.
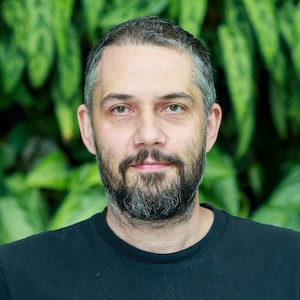
Salaries
Is it time for a new job? Check out new job offers for:
In the data science sector, where the mainstream prevails, developing niches such as AI Engineering and Prompt Engineering, which are directly driven by advances in generative artificial intelligence (Gen.AI), are noteworthy. The rise in interest in AI Engineering, reaching 19%, and the emergence of Prompt Engineering, albeit currently at 1.9%, highlight the growing demand for experts in the field of Gen.AI. This dynamic correlates with the popularization of advanced language models and AI technologies, which are becoming more accessible and integrated with daily applications. ML engineers, with a score of 23.8%, remain the foundation, enabling the development and integration of these innovations. This diversification in data science not only indicates an evolution in skills and competencies but also suggests a need for deeper specialization in response to the challenges associated with increasing data complexity and market expectations.